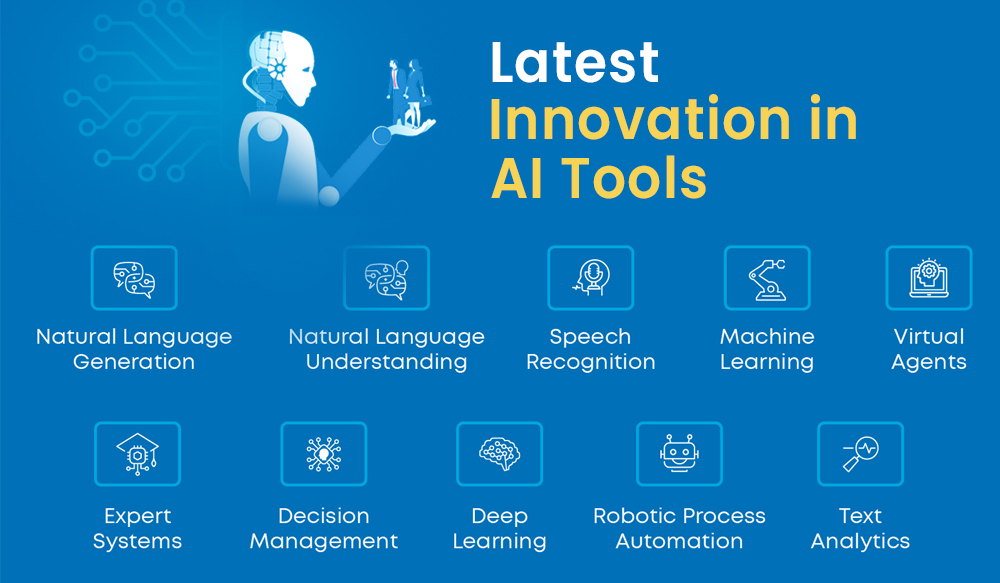
Introduction:
Artificial Intelligence (AI) has emerged as one of the most transformative technologies of our time, revolutionizing various industries and reshaping how we live and work. With each passing year, the latest innovations in artificial intelligence are pushing the boundaries of what machines can accomplish. In this article, we will explore nine secret techniques that can help improve the latest innovations in artificial intelligence. Artificial Intelligence (AI) has transformed numerous industries, revolutionizing our lives and work. AI has become integral to our daily lives, from self-driving cars to virtual assistants. As technology continues to evolve, so do the latest innovations in artificial intelligence. In this article, we will explore nine secret techniques that can be applied to enhance and improve the latest advancements in AI.
- Transfer Learning:
Transfer learning is a technique that allows AI models to leverage knowledge gained from one task and apply it to another. By pretraining models on large datasets, developers can create a foundation of knowledge that can be fine-tuned for specific tasks. This technique helps accelerate model training, improves performance, and reduces the need for extensive labelled data.
- Explainable AI:
One of the challenges in AI adoption is the need for more transparency and interpretability of AI models. Explainable AI aims to address this issue by providing insights into the decision-making process of AI systems. Techniques such as attention mechanisms, rule-based systems, and model visualization help enhance interpretability, making AI more trustworthy and accountable.
- Reinforcement Learning:
Reinforcement learning is a branch of AI that trains agents to make sequential decisions through trial and error. Recent innovations in reinforcement learning, such as model-based reinforcement learning and meta-learning, have shown remarkable progress in complex tasks like game playing, robotics, and autonomous driving. We can unlock even more significant advancements in this field by improving algorithms and exploration strategies.
- Generative Adversarial Networks (GANs):
GANs have revolutionized the field of generative modelling by enabling the creation of realistic synthetic data. These networks consist of a generator and a discriminator that compete against each other, leading to the generation of high-quality, practical outputs. Further advancements in GANs can enhance applications like image synthesis, video generation, and data augmentation.
- Edge Computing:
Edge computing involves performing AI computations closer to the data source, reducing latency and reliance on cloud infrastructure. By leveraging edge computing, AI systems can operate in real-time, enabling applications in areas with limited connectivity or strict latency requirements. Advancements in hardware and edge AI algorithms will further empower devices at the edge to perform complex AI tasks efficiently.
- Federated Learning:
Federated learning allows multiple devices to train a shared AI model collaboratively without sharing raw data. This technique addresses privacy concerns by keeping data decentralized while still benefiting from collective knowledge. By improving the efficiency, security, and scalability of federated learning; we can unlock new possibilities in areas such as healthcare, finance, and IoT.
- Human-Machine Collaboration:
The future of AI lies in the seamless collaboration between humans and machines. Innovations in human-machine collaboration involve developing AI systems that can understand and adapt to human intentions, preferences, and emotions. Natural language processing, computer vision, and affective computing are key areas where advancements can enhance human-AI interaction and create more user-centric applications.
- Quantum Computing:
Quantum computing has the potential to revolutionize AI by providing exponential computational power. Quantum machine learning algorithms and quantum neural networks are being explored to tackle complex AI problems more efficiently. As quantum hardware advances, we can expect breakthroughs in optimization, simulation, and pattern recognition tasks.
- Ethical AI:
As AI becomes more integrated into society, ethical considerations become paramount. Innovations in ethical AI focus on developing frameworks and tools that promote fairness, transparency, and accountability in AI systems. Techniques such as bias mitigation, explainability, and algorithmic auditing ensure that AI benefits all individuals and respects societal values.
- Generative Adversarial Networks (GANs) for Data Augmentation:
Data scarcity often poses a challenge in AI development. However, the advent of Generative Adversarial Networks (GANs) has opened new possibilities for data augmentation. GANs can generate synthetic data that resembles real data, allowing AI models to be trained on more diverse and abundant datasets. This technique has proven effective in improving AI models’ performance and generalization capabilities.
- Reinforcement Learning and Deep Learning Integration:
The combination of reinforcement learning and deep learning has paved the way for remarkable advancements in AI. Reinforcement learning allows AI systems to learn from interactions with their environment, while deep understanding enables them to process complex data and extract meaningful patterns. By integrating these two techniques, researchers have achieved breakthroughs in areas like robotics, gaming, and natural language processing.
- Explainable AI for Transparent Decision-Making :
The lack of transparency and interpretability has been a major concern in AI applications. Explainable AI techniques aim to address this issue by providing insights into how AI models arrive at their decisions. By incorporating explainable AI methods, such as rule-based systems and model-agnostic interpretability, we can improve trust in AI systems and ensure accountability in critical domains such as healthcare and finance.
- Federated Learning for Privacy-Preserving AI:
Data privacy is a growing concern in the age of AI. Federated Learning offers a solution by enabling AI models to be trained across multiple devices or servers without data centralization. This technique allows individuals to retain control over their data while contributing to improving AI models. We can achieve privacy-preserving AI applications by implementing federated learning without compromising performance.
- Transfer Learning for Efficient Model Training:
Training deep neural networks from scratch can be time-consuming and computationally expensive. Transfer learning addresses this challenge by leveraging pre-trained models and transferring their knowledge to new tasks. By fine-tuning pre-trained models, AI developers can significantly reduce training time and computational resources while achieving competitive performance on new domains.
- Quantum Computing for Enhanced AI Capabilities:
Quantum computing has the potential to revolutionize AI by exponentially increasing computational power. Quantum algorithms, such as quantum machine learning and quantum neural networks, can solve complex optimization problems more efficiently. As quantum computers become more accessible, leveraging their capabilities in AI research will unlock new frontiers and enable advancements in various AI applications.
- Edge Computing for Real-Time AI Processing:
Edge computing brings AI processing closer to the data source, reducing latency and enabling real-time decision-making. By deploying AI models directly on edge devices, such as smartphones or Internet of Things (IoT) devices, we can overcome the limitations of cloud computing and achieve faster response times. This technique is particularly beneficial in applications with critical low latency, such as autonomous vehicles and healthcare monitoring.
Conclusion:
The latest innovations in artificial intelligence are propelling us towards a future where machines can perform increasingly complex tasks. Collaborate with humans, and solve critical problems. By applying the nine secret techniques mentioned in this article – transfer learning, explainable AI, reinforcement learning, GANs, edge computing, federated learning, human-machine collaboration, quantum computing, and ethical AI – we can unlock the full potential of AI and drive its advancements even further. As we navigate this AI-driven world, we must ensure that these technologies are developed responsibly, with a focus on the greater good and a commitment to ethical practices.
Artificial Intelligence continues to evolve, and the latest innovations hold immense potential for transforming industries and improving our lives. By applying the secret techniques outlined in this article, such as reinforcement learning and deep learning integration, explainable AI, and quantum computing, we can push the boundaries of AI research and create more efficient, transparent, and inclusive AI systems. Embracing these techniques will enable us to unlock the full potential of AI and shape a future where technology works hand-in-hand with human needs.